Everything to Know about Web Recommendation
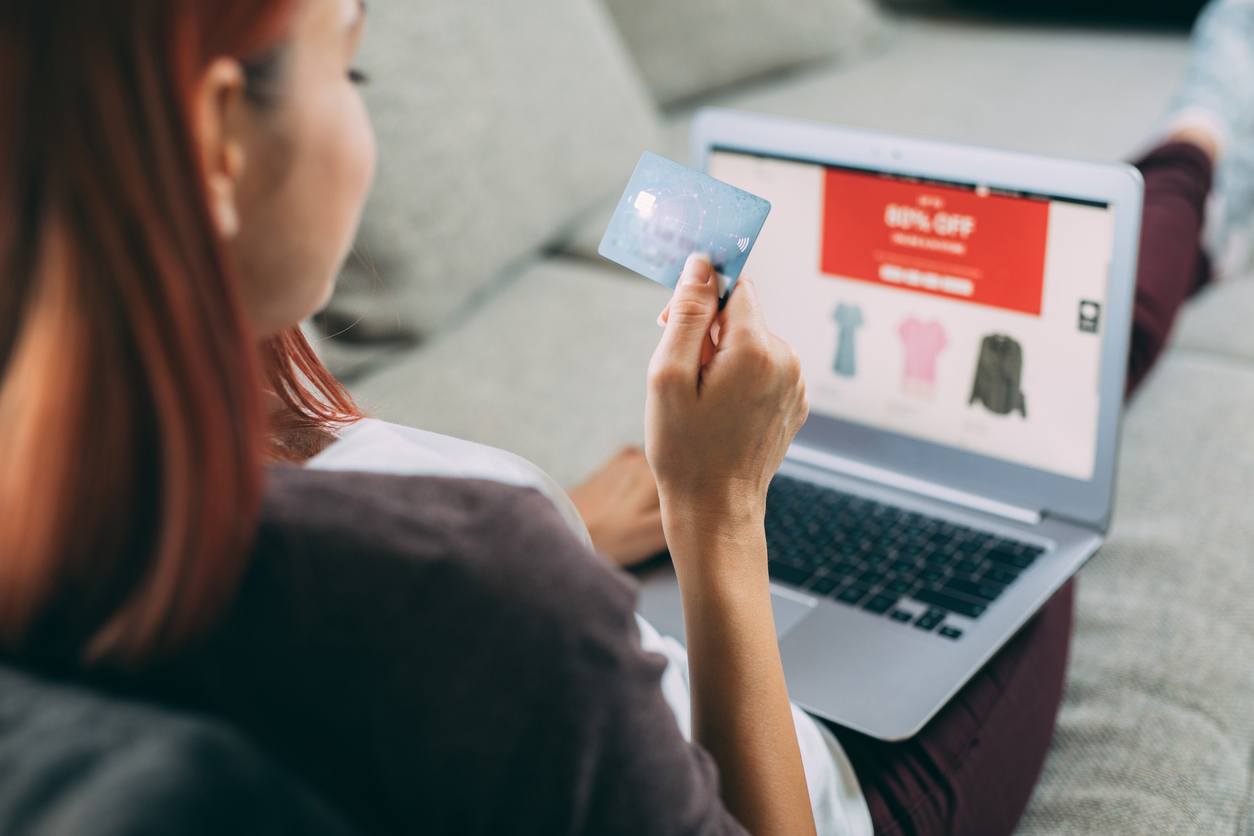
Absent the ability to inspect an item in-person, many customers turn to recommendations to research and make purchasing decisions. And they’re a popular source for product recommendation and research: 36% of shoppers age 25-34 rely on brand and product reviews before making a purchase.
But while reviews remain an important factor in shopping, faith in them has diminished since a series of high-profile fake-review scams: even Amazon is struggling to purge fake reviews and ratings from its store. But there’s more to web recommendation than customer reviews. Web recommendation algorithms use AI to suggest products, content and services that users are likely to enjoy, requiring input only from the end user (such as recommending based on their own, not other users’, ratings).
What is Web Recommendation?
The online recommendation engine definition is this: recommendation systems sort data through filtering and algorithms to make a recommendation to a user. Recommendation engine algorithms work to provide products that are personalized for the user (“items perfect for new moms,” for example) or group together related products. Data that powers a recommendation system includes explicit data (product ratings, reviews, surveys, etc.) and implicit data based on user behaviour (CTRs, purchase history, cart events and more).
The online recommendation engine definition includes two different kinds of web recommendation: collaborative filtering and content-based filtering. Collaborative filtering draws data from user behaviours to find similarities between customers or products. User-user collaborative filtering will draw comparisons between customers’ interests by using their purchase history: if you bought Items A and B and someone else bought A, B and C, you might be interested in buying C, too. Item-item collaborative filtering finds related items between products based on views. You’ve likely seen a “Customers who have viewed this item have also viewed…” recommendation engine on eBay or Amazon, which uses collaborative filtering.
Another type of recommendation engine algorithm is content-based filtering. This web recommendation system assumes that if a customer enjoyed one product or piece of media, they are likely to enjoy another. It’s not tied to behaviour, but instead pairs content by similarities in defined, descriptive keywords.
Some web recommendations might combine the two types. For example, Netflix groups its content into hyper-specific genres (content-based filtering), then provides a rating on how likely a user will like it based on their ratings of previously viewed content (collaborative filtering).
Why—and How—Should You Use Web Recommendation?
A good web recommendation system helps customers find the perfect products, services and brands for their needs. By curating like-items every time customers research a purchase, they help fight against information overload. When web recommendation systems show customers a product that’s perfect for them that they didn’t even know existed, it also adds a little delight to the customer journey. Web recommendation improves CTRs as well by providing the user with content they’re likely to take interest in.
Grouping items through recommendation engine algorithms are great for cross- and up-selling purchases as well. This is where a web recommendation engine closely resembles a shop assistant at a brick-and-mortar store. For example, if a customer is shopping for leather shoes, the recommendation engine might also suggest they purchase special leather conditioner and cleaners as well. While your business can’t possibly speak individually to every customer browsing your store, you can still provide great, personalized service with web recommendation engine algorithms.
So, how are retailers and marketers using web recommendation engines to improve their customer experience? One use case for web recommendation engines is increasing retention. This is an ideal strategy for media platforms that want to keep users engaged as long and as often as possible. Spotify and Netflix use web recommendation to frame their services as discovery platforms—for example, Spotify’s daily personalized playlist for each individual user. When Netflix found that users get bored after 60-90 seconds of choosing a show or movie, they focused on improving their recommendation engine to keep them from unsubscribing—an engine that’s now worth over $1 billion per year.
Web recommendation is also used to increase delight in the user experience. Whether it’s a fascinating product that the customer is discovering themselves or a novel piece of content, recommendation engines are great at making unexpected recommendations. YouTube does this with its Home tab and list of related videos.
Most retailers, though, use recommendation systems to increase cart value. In addition to reducing the number of abandoned carts, more accurate product recommendation may increase cart value through cross-selling. Amazon’s web recommendation system does a great job of encouraging users to add more items to their shipment with every purchase. A whopping 35% of Amazon’s revenue comes from cross- and up-selling alone, and they have several strategies to do this: grouping items commonly purchased together, recommendations based on items users own already, comparing similar items and showing what similar customers have bought.
Key Benefits for Using Recommendation Engine Algorithms
In short, web recommendation engines boost revenue by increasing customer satisfaction and retention. Through filtering both implicit and explicit user data, web recommendation offers improved personalization and irresistible offers. Finally, reporting on this user data and customer preferences can help you devise new campaigns, improve your messaging to various segments and design whole new services tailored to emerging or previously undiscovered demographics.